Manolis Zampetakis - Research Focus
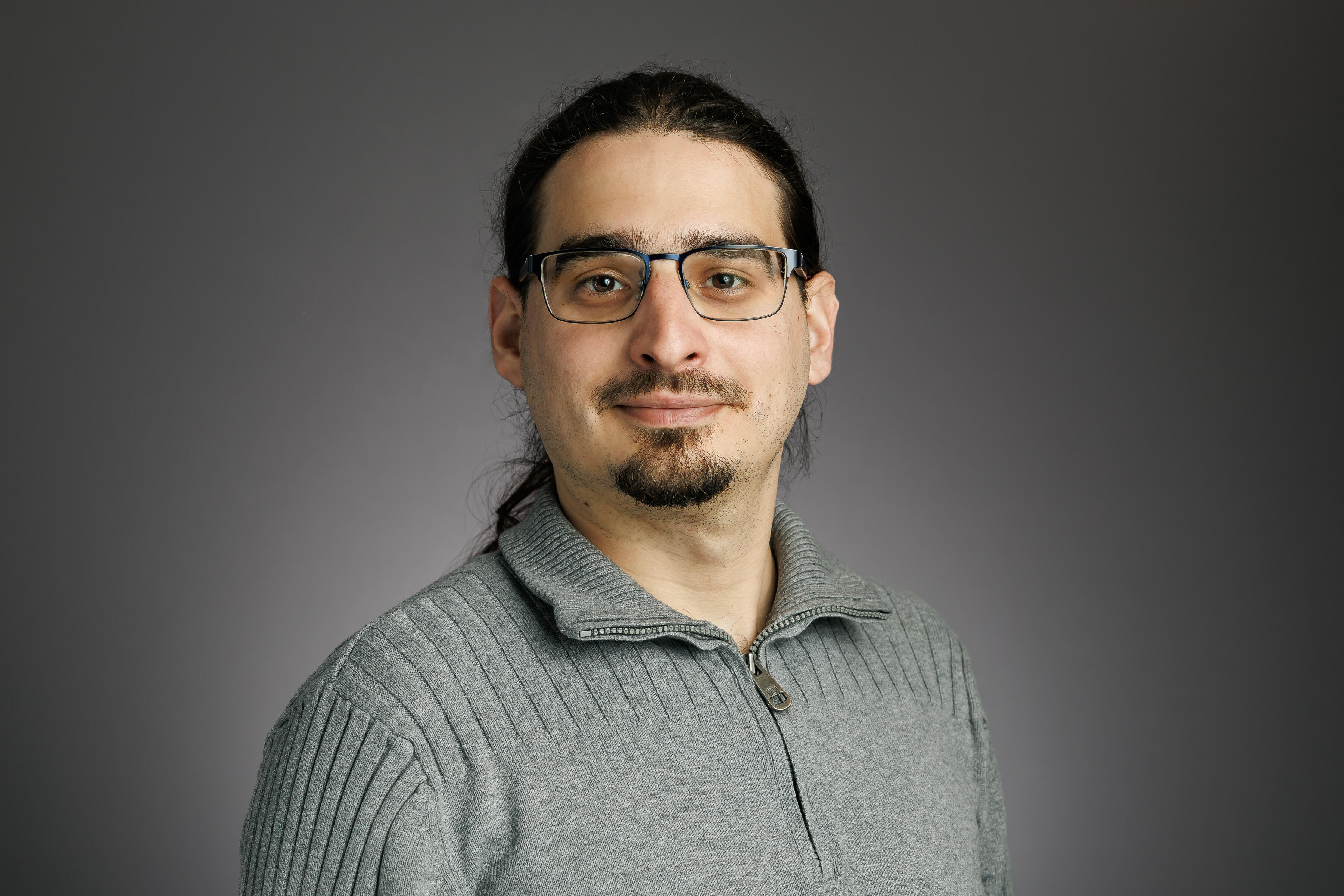
Manolis Zampetakis - Faculty Fellow
Assistant Professor of Computer Science - Yale University
-
Systematic Bias. The last few years we have witnessed a lot of cases of biased or unfair of machine learning systems. One, among many, of the reasons for this behavior is the presence of bias or corruption in the data can lead to statistical conclusions that are fallacious or unfair. As a result, identifying the sources of bias or corruption, and most importantly developing ways to perform statistical analysis even in the presence of bias is a fundamental problem with many applications in a wide range of scientific areas, including econometrics. Our focus is on bias that is the same of similar across the whole dataset, which we call systematic bias. Two popular examples of systematic bias are: truncation bias and self-selection bias. Truncation bias occurs when data falling outside of a subset of the population are not observable, and self-selection bias occurs when, for every data point, only one of the attributes is observed according to a self-selection rule. Such phenomena have been known to affect data analysis in counterintuitive ways, and dealing with them is a core problem in statistics and econometrics since at least the 1950s. We provide provably, time and sample, efficient methods for accomplishing fundamental statistical tasks in the presence of truncation or self-selection bias.
Multi-Agent Environments. To increase the social value of ML systems, we need to consider the existence of multiple agents that might be strategic and have different, many times opposing, objectives. We have provided fundamental limitations for two-agent settings with opposite objectives, namely min-max optimization, compared to the single objective ones. We have also provided new algorithms and theoretical frameworks to deal with the fundamental instantiations of multi-agent ML, as well as theoretical tools for robust decision making in the presence of strategic behavior.